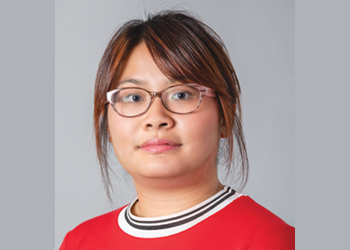
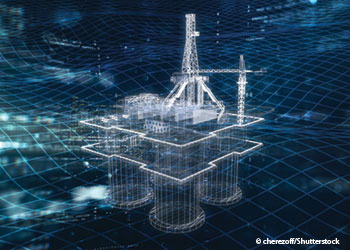
CBM has the potential to deliver data-driven value to offshore operations on two major conditions: that the data leveraged is of high quality, and that decision makers are willing to accept risk, Subrat Nanda, Chief Data Scientist, ABS, tells OGN
Most of us will have come across the saying ‘data is the new oil’. Although this term is becoming something of a loosely-used buzzword in industrial circles, it is gaining traction for good reason.
"I have been working in the applied and industrial artificial intelligence (AI) field for the best part of 20 years. To me, data turned into insights represents the bridge between theory and real-life practice. It helps us to measure and solve uncertainty and test assumptions against reality. It provides a non-biased, repeatable and factual grounding from which we can transform for the better," says Subrat Nanda, Chief Data Scientist, American Bureau of Shipping (ABS).
In the offshore industry, we are currently sitting at the tip of an iceberg in regard to unlocking the potential held inside unstructured data. If leveraged effectively, these data driven insights can inform decisions across an enormous scope of critical business functions – from improved operations and informed planning to focused training and personnel development. The potential to advance safety performance is also significant.
Unplanned downtime, as we know, is costly for all offshore operators. A study by Baker Hughes found that 1 per cent of unplanned downtime (3.65 days a year) costs offshore oil and gas organisations on average $5.037 million annually. The industry averages a little over 27 days of downtime every 12 months, which translates into costs of about $38 million. For the worst performers, figures are upwards of $88 million.
WHY QUALITY DATA MATTERS
Predictive maintenance, which anticipates problems and enables them to be fixed before they arise, is a critical part of the solution.
Similarly, condition-based maintenance (CBM) is a maintenance strategy that dictates decisions about what work needs to be carried out based on the actual condition of an asset.
![]() |
Nanda ... turning data into insights |
Under CBM, maintenance should only be performed when certain indicators are triggered and when it is economically optimal. In other words, when there are signs of decreasing performance and/or upcoming failures and determining the right opportunity to perform maintenance at an economically optimal time or location.
The need for CBM arises in part due to the challenges of time-based maintenance practices, as well as reducing uncertainty during maintenance events, requirements to safely extend the service life of equipment and achieve maximum availability.
Indeed, the evolution of maintenance strategy has followed the path from corrective maintenance to preventive maintenance to predictive and CBM; each evolution helping to take away more uncertainties from decision makers while maintaining safety standards.
It is important to stress, however, that CBM is not a replacement for subject matter experts. In fact, CBM relies on their input to train and utilise their experiential knowledge to guide improvements. Rather, it is a methodology and not a tool designed to inform maintenance strategies.
And such information is only valuable if the data fueling CBM is of high quality. This is where data science comes in.
Recent developments in this field are enabling new opportunities for marine and offshore operators to adopt a more effective asset management strategy, the crux of this strategy being to combine data analytics with historical data and operational experience to reduce unplanned downtime and achieve higher operational availability.
This involves fusing data generated from operations and prior maintenance, covering diverse datasets from sources such as equipment design information, sensor time series data, maintenance records, inspection records, performance reports and class-survey reports.
From this, an understanding of observed failure trends and risks can be gained, which in turn provides the data-driven insights needed to underpin CBM.
One of the largest obstacles to obtaining maximum value from this exercise is that the maintenance history and operator observational data is typically unstructured. This limits the achievement of CBM insights to be based mostly on structured parameter sensor data and in-situ or offline tests such as vibration and oil quality.
In response to this problem, part of my recent work has been looking at ways to better unlock the value of unstructured data, usually stored in an operator’s computerised maintenance management system (CMMS), repair and spare logs and other repositories where unstructured data gets generated in an operation.
A typical offshore CMMS allows users to input free-text status reports and many of the drop-down fields often have missing or incomplete entries. The problem with free-text fields is that they are written in a natural language format, making it a major contributor to the poor quality of data generally found in a CMMS.
Specific examples of data quality issues include: non-standard abbreviations used by different operators and crew; inconsistent equipment taxonomy; leaving critical CMMS fields blank due to lack of time or knowledge; common spelling and grammar mistakes; variation in sentence structures used to describe the same situation, etc.
All of this means that datasets must be analysed to extract useful information locked in an unstructured form before further analyses can be performed – a process which can be the difference between uncovering a systemic problem and letting it slip through the net.
Historical maintenance records have been used to train AI algorithms capable of working with unstructured free-form data to automate the task of identifying maintenance action types, differentiating maintenance scope and also isolating maintenance scope. This results in faster, repeatable and more accurate analysis leading into identifying emergent issues and model the reliability risks in an asset fleet.
This formed a key part of our recent studies, which involved developing advanced methods to perform natural language processing customised to the unique problem domain of marine maintenance.
We concentrated efforts on measuring, identifying and improving data quality issues. This involved building models to perform automated annotation via various classification methods, and several models using different hypothesis spaces and relative strengths were tested for building model ensembles. These included randomisation-based methods, kernel-based techniques, probabilistic models and instance based learning ideas.
Following this work, we now have generalisable, accurate and automated modelling process to extract insights from otherwise unstructured and free text information, coming from the domain of diverse marine and offshore assets. In so doing, we have also developed a set of artificial intelligence methods to address the data quality challenge posed by unstructured operations generated data sources.
This presents many advantages. First, it facilitates faster and more reliable data processing, and provides robustness against variations in expression of semantics which are commonplace in marine and offshore working environments.
Furthermore, it grants the ability to perform data fusion, treating multiple sources of data as one as opposed to in siloes.
TRUST THE DATA
By adopting some or all of these measures to enhance data quality and derive maximum effectiveness of condition-based maintenance, offshore operators could reverse much of the disruption and financial cost caused by unplanned downtime.
Furthermore, quality maintenance data (and thus improved analytics over time) can inform other fundamental business operations such as human development and training. For OEMs, it can highlight common faults, which can be fixed at source on the production line before it even reaches an end-user, or identification of equipment issues before they go on to become widespread across the deployed fleet, prompting timely design, controls or configuration enhancement.
At the same time, however, the added value brought to offshore maintenance strategies by data science must also be met with a willingness to make risk-based decisions and embrace changes.
As well as deepening collaboration between offshore operators, class societies such as ABS and OEMs towards a standardised equipment hierarchy, the industry needs to adopt a more condition-based paradigm to enable optimised decision making. Buy-in from business leaders is fundamental if maximum value from data is to be extracted.
But this is not just a one-way conversation. We as data scientists must also accept that we must do a better job of applying our findings to live situations. It is, therefore, upon us data scientists to be cognisant of problems such as overfitting, confusing correlation for causation and over-estimating the ability of our models to generalise. The onus should also be on us to communicate this clearly to offshore decision makers who operate in the real world.
Indeed, it will need to be a collaborative effort between various stakeholders. The industry needs to start using insights from data-driven programs, with SMEs and operators playing their part of the algorithm building process with the data scientists. This will only improve trust and adoption.
Arguably the most important input, however, will come from the top. Utilising and, critically, acting upon data-driven condition-based recommendations requires deliberate top-down support from executive leadership. Data and AI must become part of the C-suite realm if a real, lasting impact is to be made.