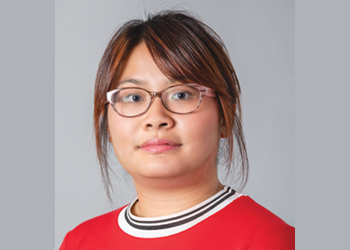
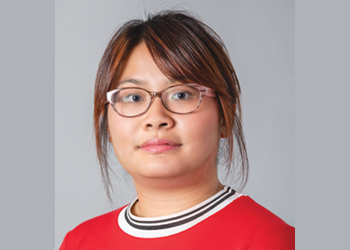
High quality input data is required to realise the benefits of using advanced modelling techniques, and this means having a well maintained data system and a better understanding of the data itself, Dr Yanfeng Liang from TÜV SÜD National Engineering Laboratory tells OGN
In A world where data is output at high speeds and stored in large volumes, new challenges must be met, such as datasets containing large numbers of variables output at high frequencies (high dimensionality data), inconsistency in data labelling and poorly structured databases.
It is becoming ever more apparent that in order to maximise the value of diagnostic information stored within said data, we must make use of advanced modelling techniques, for example, machine learning models.
A data analytic algorithm that teaches computers to perform tasks by learning from experience, a machine learning (ML) model can either be categorised as ‘supervised learning’ or ‘unsupervised learning’.
Both have their advantages and disadvantages and the deciding factor on which model to use is highly dependent on the type of data and the type of question that one wishes to address. A more detailed comparison between a supervised model and an unsupervised model is given in Table 1.
![]() |
In flow measurement, ultrasonic flow meters (USMs) are capable of outputting a large number of digital process variables which can provide information pertaining to meter health and process conditions. Device measurement error can manifest as drifts within these ‘diagnostic’ variables. However, different process conditions can evoke the same diagnostic variable drift and therefore create ambiguity for the end-user tasked with interpreting the data.
ML models can be used to overcome this ambiguity as well as extracting the most important variables in determining a specific operating condition. This reduces the risk of including redundant and misleading data which could jeopardise the accuracy of the model as well as pinpointing to end-users the key variables to monitor changes.
CASE STUDY
The following case study illustrates the capability and advantages of using ML models and in selecting the most important variables. It was done to detecting the presence of an unwanted second phase in fluid using ML models.
The presence of an unwanted second phase in a single-phase fluid affects the accuracy and the reliability of a flowmeter’s output data. To enhance our understanding on this, a project was undertaken by TÜV SÜD National Engineering Laboratory where varying percentages of gas (gas volume fractions ranging from 0 per cent GVF to 10 per cent GVF) were deliberately injected into the fluid in an effort to investigate the subsequent effects on meter performance.
Different ultrasonic flow meters (USMs) — referred to here as USM A — were tested in the project and this article shows the prediction results related to one of the USMs are given (Figure 1).
As the data was labelled, a supervised ML learning model was built based on the experimental data.
![]() |
The experimental data consisted of 55 variables with 11,081 observations, where the data was further divided into training data, validation data and unseen data. The training data was used to teach the model to learn the expected patterns and interrelationships in variables when exposed to different percentages of gas. The model’s prediction capability was then tested using the validation data.
Based on the training and validation data, the model achieved an average accuracy rate of 98.62 per cent in assigning the data to the right gas classes. Groups of unseen data, not used during the training and the validation stages of the model, were set aside and, used to further test the model’s prediction ability.
For example, for unseen data C, the model correctly predicted 90.42 per cent of the data, attributing those to the condition of having 1.5 per cent GVF in the fluid. For the remaining 9.58 per cent, the model falsely predicted that those belong to other GVF groups. Therefore, this set of data was likely to be gathered when a fraction of unwanted second-phase (gas) was present within the system.
The results on other unseen data can be interpreted in a similar manner. It is promising to see that the model had classified data in different GVF classes with high accuracy, by finding hidden patterns and correlations between variables.
Results such as these would be beneficial to end-users who wish to identify how much gas is present within the fluid based on drifts experienced in certain variables. From an end-user perspective, having the ability to predict the percentage of gas present within the water and thus the degree of effect on the performance of USMs can aid in decision-making and maintenance processes.
AFFECTED VARIABLES BY PRESENCE OF GAS
The data used contained the output of 55 different variables from the USM, and each variable has multiple interrelationships with others. Some of these variables are crucial in determining the health and performance of the flowmeters, whilst some could be misleading and redundant in identifying the presence of gas within the system.
How do we decide which variables to neglect for different operating conditions? How can end-users, who do not possess the necessary working knowledge, decide which variables are important? These questions can be answered through the use of a supervised machine learning model. After learning the patterns and correlations between variables, the model has the capability to pinpoint which variables play the most crucial role in identifying the stated error.
![]() |
For the case study described, the model detected the variables given in Figure 2 to be important, where the most important variable is ranked at the top. In this case, the sound velocity (SndVel) for different chords in USM A along with their averaged values (AvgSndVel) were considered to be most affected by GVF.
The importance of the variables was determined based on how much accuracy the model lost if that variable was omitted during the prediction process; the more accuracy the model lost, the more important that variable is in determining the percentage of gas in the fluid.
Through the use of ML models and advanced modelling techniques, valuable information can be extracted from data which end-users will benefit from, allowing them to improve their decision-making and ability to diagnose faults, as illustrated by the case study here.
As the world moves further towards digitalisation, more data is being produced than ever before. However, to realise the benefits of using advanced modelling techniques and to prevent data from becoming the new plastic, high quality input data is needed.
This requires end-users to have a well maintained and structured data system and a better understanding of the meanings that lie within the data. Input from industry experts is also needed to account for human experience in specific scenarios to optimise the information that can be extracted from data.
Dr Yanfeng Liang is a researcher and mathematician at the TÜV SÜD National Engineering Laboratory. She is a member of the Institute of Mathematics and its Application, a chartered professional body for Mathematicians, and holds an honorary research associate position at the University of Strathclyde.
TÜV SÜD National Engineering Laboratory is a world-class provider of technical consultancy, research, testing and programme management services. Part of the TÜV SÜD Group, the laboratory is also a global centre of excellence for flow measurement and fluid flow systems and is the UK’s National Measurement Institute for Flow Measurement.