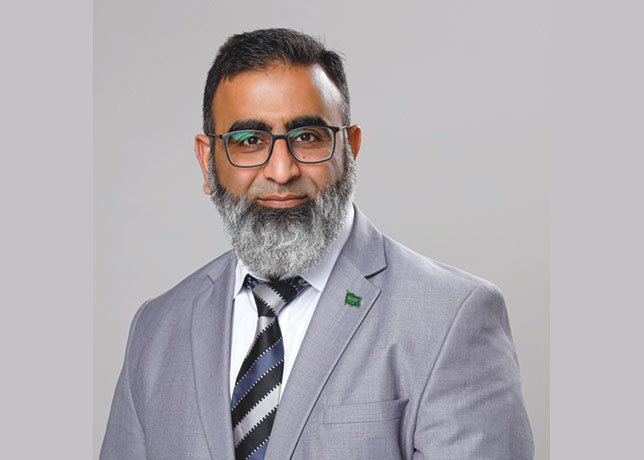
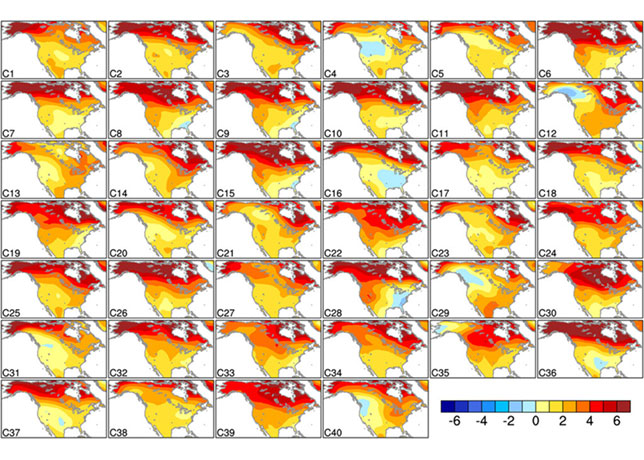
Climate models are far from perfect, but they are also incredibly informative. Most of the criticisms of models are based on misleading analyses, writes Andrew Dessler of The Climate Brink
In the world of climate communications, no claim seems to come up more frequently than 'The climate models are wrong!' Today, it’s the claim that the models are hot and future warming will be much less than they predict. The source is some internet post by a global figure (Figure 1).
First, let’s be clear: Climate models have an admirable track record of predicting the global average temperature. But the post in questions is about regional and seasonal climate change. While there are few details provided in the source document, I was able to reproduce the general result presented (Figure 2). So, does this mean that models are warming too much?
THE PROBLEM WITH REGIONAL COMPARISONS
While the global average temperature is well constrained by planetary energy balance, how that warming is distributed around the planet can vary greatly due to what we refer to as unforced variability. This phenomenon is similar to the randomness we observe in weather patterns, but are driven by the ocean circulation on much longer time scales, such as the El Niño/La Niña oscillations.
![]() |
Figure 1 ... climate models 'vastly overstated' warming |
Unforced variability can warm one region while simultaneously cooling another, with such variations spanning decades. This long-term variability adds a layer of complexity to prediction of regional climate change, even as the global average warming trend is well constrained.
As an example, you can take a climate model and run it many times, with identical emissions of carbon dioxide, but with each run starting with a slightly different state of the climate system. For these runs, the global average temperature change is similar, but how the warming is distributed varies greatly (Figure 3)**.
In some of the ensemble members (for example, C16, C28), the Southeast US cools between 2010 and 2060, while in most others it warms. In some members (for example, C4, C29, C40), the Pacific Northwest cools, while in most others it warms.
This has important implications for Derwood’s plot. To see this, let’s take a single model, in this case, ACCESS-ESM1-5, which has an ensemble of 40 historical runs in the CMIP6 archive. Each run has different initial conditions, so each run of the model will have a different pattern of warming and cooling, just like we see in the plot above.
Summertime central US from this one model ensemble looks like Figure 4.
Unforced variability has a huge impact on what this model predicts. Some 20 per cent of the runs show smaller warming than observations, and 8 per cent show cooling.
At the other end, there are ensemble members that show five times the observed warming. This spread is due entirely to different initial conditions, so this is an example of chaos in a strongly non-linear system.
![]() |
Figure 2 ... the author reproduced the general result in Figure 1 |
So, is the model overestimating warming or not? There’s no way to know. If you pick ensemble member 17, the model is underestimating the warming. If you pick ensemble member 35, the model is doing a fantastic job, while ensemble member 18 is overestimating the warming.
Without figuring out which of these ensemble members has unforced variability matching the unforced variability in the real world (this is really hard, by the way), the only correct conclusion is that the observed warming falls within the envelope of warming predicted by this model, so you cannot conclude that the ACCESS-ESM1-5 is overestimating warming.
This doesn’t mean that this model is right, of course. But it does mean that the plot in Figure 1, which shows one value for this model, about 0.6 deg C, is inaccurate.
To construct the Figure 1 plot, I’m guessing a random run of each model was selected. So, let’s remake the plot, but using the ensemble member from each model that shows the lowest warming in this region. I limit myself to models that have ensembles with, at least, five runs in the CMIP6 archive (Figure 5).
![]() |
Figure 3 ... Winter surface temperature trends, 2010–60; deg C (51 yr) from each of the 40 CCSM3 ensemble members |
This shows that the results of the ACCESS-ESM1-5 are generalisable. Two thirds of the CMIP6 models produce warming lower than observations in their ensembles.
Again, this doesn’t prove the models are right, but it does say that naive comparisons that ignore unforced variability, like Derwood’s plot, should not be taken seriously.
WHAT’S A BETTER WAY TO DO THE CALCULATION?
While unforced variability can impact any single location, if you look globally, the impact of it cancels out – regions that are cooled by unforced variability are cancelled by other regions that are warmed. Thus, the right way to do this calculation is globally.
To that end, I’ve taken a single (random) run of each of the 45 CMIP6 models and the observations and calculated this same change in summertime surface temperature, but at each point on the globe.
If the CMIP6 models are accurately simulating the climate, I would expect that areas where the model average is warming faster than the observations would be about equal to the area where the model average is warming slower.
![]() |
Figure-5 models that have ensembles with, at least, five runs in the CMIP6 archive |
In reality, the average warming in the CMIP6 models exceeds observed warming over 63 per cent of the area of the Earth. This is larger than our expected 50 per cent, but it is consistent with analyses showing that a subset of CMIP6 models does appear to be running too hot.
If we eliminate these too-hot models by screening out the models that have equilibrium climate sensitivity that’s out of the accepted range (2C-4C)1, then the average warming of the models exceeds observations over 48 per cent of the area of the Earth, with observed warming exceeding the models over the other 52 per cent.
This is exactly what we would expect if the models are accurately simulating the climate system.
WHAT’S REALLY GOING ON?
This plot in Figure 6 shows the difference between the average of the screened model ensemble and the observations.
Red colors indicate where the average model warming is faster than observations while blue areas show that models are warming slower.
As mentioned above, 52 per cent of the globe is blue, indicating places where the models are warming slower than observations.
The box over North America shows the region that the Figure 1 plot focuses on.
I have two comments on the selection of this region. First, I think it’s useful to understand the provenance of this plot. It comes from Dr Roy Spencer via the Heritage Foundation.
There are three notable points to be made. First, 14 models were analysed, but only six models were plotted and the particular observational data set that provided maximum support for their hypothesis. Plotting all of the models and all of the data provide a much different conclusion.
The other comment is that, in addition to unforced variability, this is a region where other odd things are going on. It turns out that this is the most agriculturally productive region on the planet, and land-use changes over the last few decades have largely offset greenhouse gas warming here.
To the extent that models have problems in this very small region, it’s much more likely to be connected to how well the models are handling land-use changes, not how well they represent global warming.
Thus the saying that 'all models are wrong, but some are useful' applies here – climate models are far from perfect, but they are also incredibly informative. Most of the criticisms of models are misleading analyses. This one is no exception.
* The article has been slightly revised to reflect political and other sensitivities.
** Deser et al. (2014). Projecting North American Climate over the Next 50 Years: Uncertainty due to Internal Variability. Journal of Climate. 27. 2271-2296. 10.1175/JCLI-D-13-00451.1.